How Do On-Chain Metrics Explain Bitcoin Volatility? Part 2
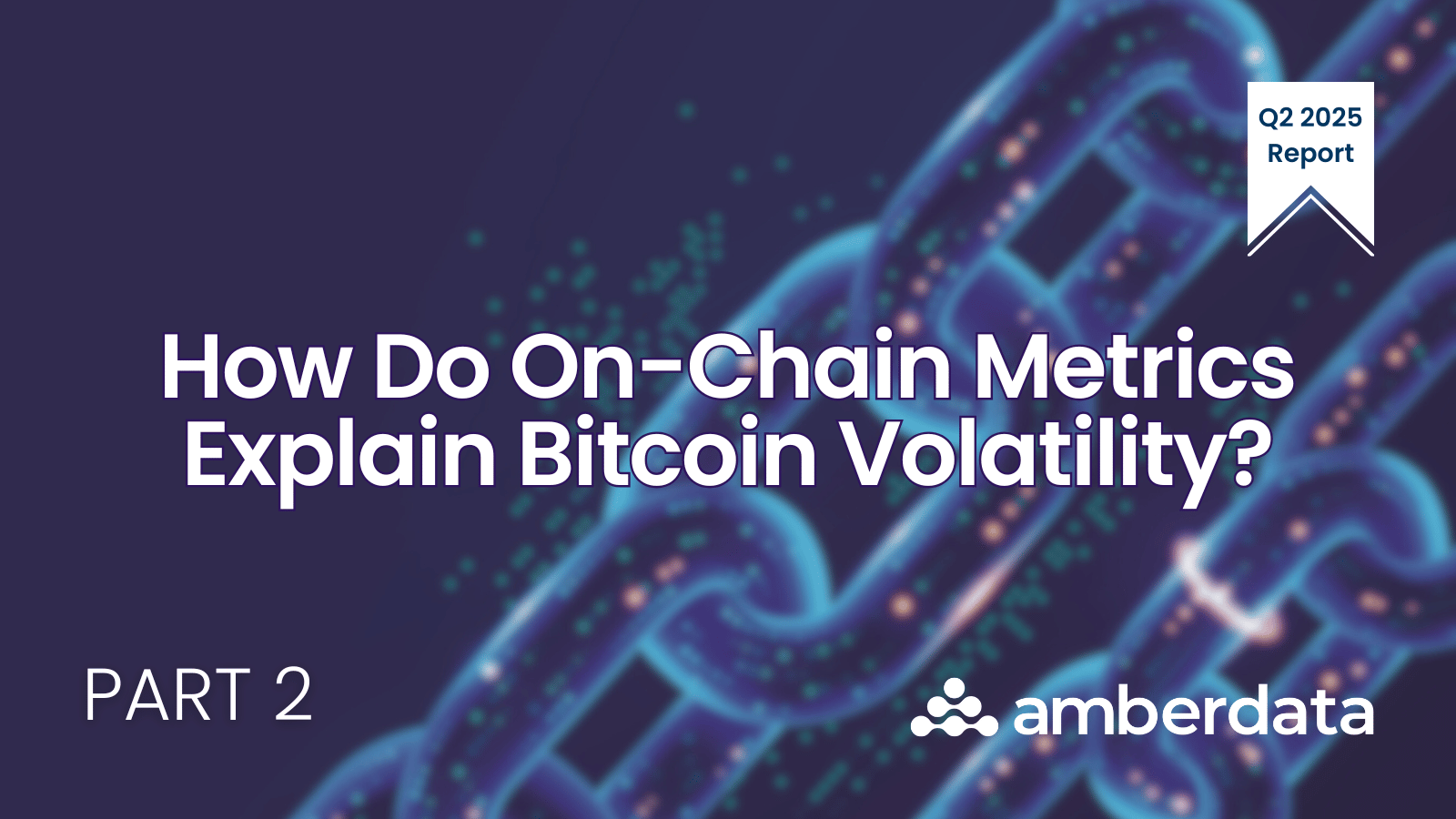
This report builds on the insights from our previous exploration, "How Do On-Chain Metrics Explain Bitcoin Volatility? Part 1" and takes a deeper dive into the mechanics driving Bitcoin’s rapid price movements. Check this out for more information on the metrics we are looking at. Expanding on earlier findings, we now scrutinize behavioral signals from a wide array of market participants—miners, institutions, short-term traders, and long-term holders—observing how these groups react before, during, and after significant volatility events we term “shock days.”
Shock days are identified when Bitcoin’s absolute daily return (ADR) exceeds two standard deviations above its historical average, capturing statistically rare and impactful price moves. We focus on two distinct market environments: bull phases (October 1, 2023, to December 16, 2024) and bear phases (November 8, 2021, to November 21, 2022), with each phase comprising exactly 21 shock days. By contrasting these opposing market conditions, we reveal how distinct on-chain metrics—both in their raw and first-differenced forms—serve as early-warning signals and markers of structural changes.
This comprehensive framework is structured around four rigorous analyses: a Lead-Lag Analysis that identifies metrics predicting impending volatility, a Pre-Shock Extremes Analysis that pinpoints when key indicators reach critical thresholds, a Mean Shift Analysis capturing the immediate behavioral shifts around shocks, and a Post-Shock Persistence Analysis assessing the durability of these changes. The resulting insights offer traders and institutions valuable predictive power and strategic advantages to anticipate market extremes, manage risk more effectively, and navigate the dynamics of Bitcoin volatility— all available directly from Amberdata.
Identifying Shock Days
To analyze Bitcoin volatility systematically, we first define clear criteria for identifying significant volatility events—termed "shock days." A volatility shock occurs on days when Bitcoin’s absolute daily return (ADR), essentially the magnitude of daily price movements regardless of direction, surpasses two standard deviations above its historical mean. This threshold effectively captures statistically rare and meaningful shifts in market sentiment and investor behavior, setting a reliable benchmark for isolating impactful volatility events.
Our study separates volatility shocks distinctly into bull and bear market environments. For bull phases, which ran from October 1, 2023, through December 16, 2024, we identified 21 such volatility shocks.
Similarly, for bear phases between November 8, 2021, and November 21, 2022, exactly 21 shock days were observed, ensuring a balanced comparative analysis across market cycles.
By categorizing these shock events according to market sentiment—bullish optimism versus bearish pessimism—we aim to explore deeper differences in investor behaviors under varying market conditions. The consistency in the number of shock days across both phases provides a robust basis for comparing and contrasting the predictive power of various on-chain signals. Traders and institutions can leverage these insights to anticipate future volatility better, potentially refining their strategies to manage risk more effectively during significant market moves.
Lead Lag Analysis
Our first major step is to identify which on-chain metrics serve as early signals for impending volatility. For this, we compute correlations between each metric and a rolling 5-day Absolute Daily Return (ADR), shifting (or “lagging”) the metrics by 1 to 14 days. In our analysis, some metrics are used in their raw form (for example, daily exchange volume or the absolute levels of daily active addresses), while others are represented as first differences (indicated by the _diff suffix, such as marketcap_diff, realizedCap_diff, and several liquidity and miner behavior metrics). This distinction means that for first-differenced variables, the lead-lag correlation reflects the day-to-day changes (i.e., the increments or decrements) rather than their absolute levels. By observing when the correlations peak, we infer how many days in advance a metric begins to signal building market pressure or momentum.
In bear markets, we find that short-term UTXO metrics—particularly the 1-day to 1-week (1d–1w) bucket—offer a consistent, early warning signal. For instance, correlation values increase steadily from as early as lag -11 and peak around lag -1 (approximately 0.49), suggesting a sustained wave of selling pressure that eventually culminates in a final burst of exits just before the shock day.
In contrast, during bull markets, short-term UTXOs exhibit only brief surges in correlation and lack the sustained pattern observed in bears. Instead, the 1-week to 1-month (1w–1m) UTXO bucket maintains a moderate-to-high correlation across the entire lag window, indicating a gradual build-up of conviction as coins “age” into this band. Additionally, non-differenced metrics—such as realized cap, along with liquidity flows to both liquid and illiquid wallets and daily exchange volume—complement these signals. In bull regimes, these metrics frequently capture profit-taking activities, whereas in bear markets, they mirror early capitulation signals. Together, these insights from both the differenced and non-differenced metrics offer traders and institutions a refined lens to anticipate volatility and adjust risk management strategies accordingly.
Pre-Shock Extremes Analysis
In this section, we examine which metrics consistently enter statistically extreme zones—defined as exceeding the 90th percentile or dropping below the 10th percentile—in the days preceding a volatility shock. Some metrics are used in their raw form (such as daily_total_exchange_volume), while others are first differences (e.g., marketcap_diff, realizedCap_diff, and various liquidity and user activity metrics). This distinction matters because first differences capture day-to-day changes rather than absolute levels.
Based on our lead-lag analysis, we set different observation windows for each market regime: 7 days for bull markets and 14 days for bear markets. We further smooth the data with a 3-day rolling average for bulls and a 5-day rolling average for bears to mitigate noise. These extreme movements—whether a spike in volume or a steep drop in net flows—offer actionable early-warning signals. They help traders track specific, quantifiable thresholds that have historically preceded significant price swings.
Bull Phase Insights
In bull markets, the pre-shock environment is marked by robust trading activity and liquidity repositioning. For example, the daily total exchange volume exceeds its 90th percentile in 14 out of 21 shock days—signaling that high-volume trading activity is a consistent precursor to volatility in bullish conditions. Similarly, realizedCap_diff records extreme high values in 12 shock days, suggesting that profit-taking (reflected by changes in realized cap) intensifies before major price moves. Additional signals include net_flows_liquid, which shows values above the 90th percentile on 11 shock days, along with short-term UTXO metrics such as utxoValue1d1w and utxoValueUnder1d, both of which also breach this upper threshold on 11 occasions.
Conversely, several metrics indicate a contraction in long-term or structural support. The first-differenced 365DayNewAddressMa_diff falls below the 10th percentile in a striking 16 out of 21 shock days, signaling that new user adoption or sustained network growth is stalling just before a shock. Furthermore, longer-term UTXO measures—utxoValue5y8y_diff and utxoValue18m24m_diff—each drop below the 10th percentile on 9 shock days, while passiveAddresses and capitulationIndex register these low extremes on 8 and 7 shock days respectively. This divergence suggests that while active trading surges, key indicators of long-term network robustness and user engagement weaken, potentially exacerbating the impact of ensuing volatility.
Bear Phase Insights
The bear market pre-shock landscape tells a different story. Here, certain metrics surge into extreme territory even as overall market activity is subdued. For instance, the first-differenced hodl_liveliness_diff exceeds the 90th percentile in 8 out of 21 shock days, pointing to an anomalous spike in short-term holder activity and perhaps an abrupt reallocation of positions amid mounting stress. Metrics such as net_flows_illiquid_diff, 365DayNewAddressMa_diff, and nupl_diff each cross above the 90th percentile in 5 shock events, while capitulationIndex does so in 4 events—indicating that even under bearish conditions, there are bursts of activity that could be interpreted as capitulatory or signal a last-minute rush to reposition.
On the downside, liquidity and trading signals reveal a pronounced fragility. Notably, net_flows_liquid (in its raw form) falls below the 10th percentile in 15 out of 21 shock days, reflecting a widespread withdrawal from liquid positions well before shocks occur. This is complemented by the short-term UTXO metric utxoValue1d1w, which dips below the 10th percentile on 9 shock days, and utxoValue1w1m, which does so on 8 days. Additionally, daily_total_exchange_volume—another non-differenced indicator—falls below its extreme threshold in 8 shock events, while hodlNetPositionChangeDaily drops below the 10th percentile in 7 events. Together, these bear-phase signals emphasize that reduced liquidity combined with declining trading activity creates a precarious environment that can amplify the subsequent impact of volatility shocks.
Mean Shift Analysis
Mean Shift Analysis focuses on the immediate aftermath of a volatility shock by comparing the average value of each metric in the 4-day window before the shock to that in the 4-day window after the shock. For metrics in their raw form, this comparison reflects changes in absolute levels, while for first-differenced metrics (identified by the _diff suffix), the analysis captures shifts in day-to-day changes. Because we are looking at relative changes—how much the “change” itself increases or decreases—the resulting mean shifts can yield large percentage differences. It is important to interpret these percentages in a relative sense: they signal a strong behavioral shift rather than a direct “growth rate” on an absolute basis.
By averaging these percentage changes across all shock days within a given market phase, we capture consistent tendencies in participant behavior immediately after significant price moves. In bull phases, for instance, increases in realizedCap_diff may signal that traders are aggressively repositioning and transacting at higher cost bases, whereas in bear phases, a marked rise in minerPositionIndex could indicate pressure on mining operations to liquidate positions. Whether these shifts are transient or more persistent, they reveal where the strongest reflex responses occur. For traders, the insights gained from these post-shock moves can inform short-term tactical decisions, such as scalping, arbitrage, or rapid portfolio rebalancing.
Bull Phase Insights
During bull phases, our analysis reveals several striking post-shock changes. On average, realizedCap_diff registers a positive shift of 171.9%, indicating a substantial increase in profit-taking or capital reallocation as market participants transact at higher realized cost bases. Concurrently, net_flows_highly_liquid_diff rises by 121%, suggesting that traders are rapidly shifting coins into highly liquid wallets to be ready for subsequent moves. Additionally, hodl_liveliness_diff increases by 82.7%, reflecting a renewed intensity among holders as they adjust their positions. User activity, as measured by 30DayNewAddressMa_diff and 365DayNewAddressMa_diff, also picks up—with increases of 73.4% and 56.86% respectively—potentially indicating a burst of engagement following the shock.
On the other hand, several metrics display pronounced negative shifts. The long-term UTXO metric utxoValue5y8y_diff plunges by 616.2%, while activeAddresses_diff drops by 280.91%, utxoValue2y3y_diff by 235.65%, and utxoValue3y5y_diff by 200.74%. In addition, the minerPositionIndex falls by 137.6%, indicating that both long-term holders and even miners may be exiting positions in response to the volatility. These figures should be viewed as indicators of relative change; the large percentages highlight a major restructuring of on-chain behaviors rather than a direct measure of the absolute amounts traded or held.
Bear Phase Insights
In bear markets, the post-shock dynamics reveal a different but equally dramatic reordering of on-chain behaviors. On the positive side, net_flows_highly_liquid_diff surges by an average of 1047.27%, clearly signaling that traders are rapidly reallocating assets into highly liquid positions in anticipation of further downturns or shorting opportunities. The 30DayNewAddressMa_diff increases by 823.88%, and the 365DayNewAddressMa_diff by 144.1%, reflecting a burst of network activity despite overall market stress. Importantly, the minerPositionIndex rises by 454.17%—a reversal compared to bull conditions—signaling that mining operations are shifting from holding to actively liquidating positions under pressure. Additionally, btc_etf_daily_flow improves by 75%, which may suggest some institutional repositioning in a bearish environment.
However, these bullish-style signals are counterbalanced by significant negative shifts. The allAddresses_diff metric falls by 355.6%, pointing to a broad contraction in network participation. Furthermore, utxoValue3y5y_diff decreases by 239.45% and utxoValue5y8y_diff by 102.16%, indicating that long-term holders are offloading their positions. RealizedCap_diff drops by 88.34%, and hodlNetPositionChangeDaily declines by 79.1%, reinforcing the overall picture of capitulation and defensive repositioning. Like in the bull phase, these large percentage changes should be interpreted as relative indicators of shifts in behavior—demonstrating how dramatically the market participants’ daily change patterns adjust in response to shocks, rather than suggesting an absolute change in asset levels.
Post-Shock Persistence Analysis
Post Shock Persistence Analysis examines how long key metrics remain meaningfully above or below their pre-shock averages. Using ±20% thresholds around the pre-shock baseline, we count the number of consecutive days (up to a maximum of 14) that each metric stays outside of this normal range. When a metric frequently remains in an elevated or depressed state post-shock, it signals a deeper behavioral or structural shift rather than just a transient reaction. Because this analysis compares changes versus changes (or the persistence of a shift in the first-differenced data), the resulting averages may seem large, but they should be interpreted as indicators of how long the market participants’ revised behavior lingers relative to their pre-shock state.
By examining all shocks within each market phase, we identify robust patterns in wallet flows, UTXO cohorts, miner behavior, and user engagement. These patterns help traders differentiate between fleeting, opportunistic moves and more fundamental regime shifts. A metric that remains outside its baseline for several consecutive days, or repeatedly across many shocks, becomes a powerful directional cue.
Bull Phase Insights
In bull markets, several metrics indicate a persistent restructuring of on-chain behavior following a shock. One standout finding is the UTXO bucket representing coins held for 1 week to 1 month (utxoValue1w1m), which remains above the 20% threshold for an average of 10.06 days in about 33% of shock events. This long duration suggests ongoing consolidation, where traders hold on to coins in this mid-range cohort, reflecting sustained optimism and speculative positioning. Similarly, the 30DayNewAddressMa_diff persists above baseline for 5.67 days on average in 71% of shocks, implying that bursts of network activity or engagement last well into the post-shock phase. RealizedCap_diff also remains elevated for 6.64 days in nearly half (48%) of the events, indicating that higher valuation dynamics—possibly reflecting profit-taking at elevated cost bases—continue for several days.
Conversely, several metrics show a sustained negative shift. The 365DayNewAddressMa_diff falls below its pre-shock baseline for an average of 10.56 days in 86% of shocks, which flags a prolonged slowdown in new address growth. Additionally, minerPositionIndex remains depressed for 8.31 days on average in 76% of shocks, while utxoValue3y5y_diff stays below threshold for 7.76 days in 71% of shock events. These results suggest that after a volatility shock in bull markets, while certain speculative activities continue, there is a notable and sustained reduction in structural growth and long-term engagement, which traders should monitor to gauge potential overextensions or cooling trends.
Bear Phase Insights
In bear markets, the post-shock persistence analysis uncovers a markedly prolonged reaction in several key metrics. On the upside, btc_etf_daily_flow is particularly persistent—remaining above its threshold for an average of 11.33 consecutive days in 100% of shock events. This uniform response points to consistent institutional or fund-based repositioning and hedging behavior during periods of market stress. Furthermore, the 365DayNewAddressMa_diff stays above the threshold for an average of 11.00 days in 67% of shocks, signaling a prolonged stagnation or even contraction in new address growth. The utxoValue6m12m_diff metric remains elevated for 5.65 days in 81% of shocks, while the 30DayNewAddressMa_diff is above threshold for 4.21 days in 67% of cases. Additionally, hodlNetPositionChangeDaily remains elevated for 4.15 days on average in 56% of shocks, suggesting sustained shifts in how long-term holders manage their positions.
On the downside, several metrics in bear markets demonstrate extended periods of decline. RealizedCap_diff stays below its pre-shock level for an average of 6.25 days in 76% of shock events, indicating that the market struggles to revert to pre-shock price levels after sell-offs. Net_flows_illiquid_diff remains depressed for 5.60 days in 33% of cases, and minerPositionIndex stays below baseline for 5.33 days in 67% of shocks, reinforcing the idea that miners are refraining from selling and contributing to persistent market pressure. Additionally, daily_total_exchange_volume is lower than its baseline for about 4.67 days in 33% of shocks, while hodl_liveliness_diff stays depressed for 4.62 days in 62% of the events. This combination of sustained low liquidity and ongoing outflows from long-term holders paints a picture of a market under prolonged stress, where the recovery process may be slow and uneven.
Conclusion
Bitcoin’s volatility, driven by rapid price swings and distinct behavioral patterns from miners, institutions, short-term traders, and long-term holders, reveals an intricate interplay between market sentiment and on-chain dynamics. Our analysis defined “shock days” as instances where Bitcoin’s absolute daily return exceeds two standard deviations above its historical average—providing a robust framework by evenly sampling 21 such days in both bull and bear phases. In our Lead-Lag Analysis, we uncovered that short-term UTXO metrics offer early signals of impending volatility: in bear markets, these signals are sustained—peaking around lag –1 with correlations near 0.49—while in bull markets, the 1w–1m UTXO bucket indicates a gradual build-up of conviction.
The Pre-Shock Extremes Analysis demonstrated that, in bullish conditions, metrics such as daily exchange volume and realizedCap_diff consistently breach upper thresholds (in 14 and 12 of 21 shock events, respectively), whereas key long-term indicators like the 365DayNewAddressMa_diff often dip below critical lows. In contrast, bear phases exhibit pronounced liquidity withdrawals and shifting holder behaviors, with net_flows_liquid falling below the 10th percentile on 15 shock days, underscoring mounting market stress.
Our Mean Shift and Post Shock Persistence Analyses further quantified both the immediate and longer-lasting shifts in market behavior. Although the resulting percentage changes are sometimes extremely large, they should be interpreted as indicators of relative changes in market dynamics—not absolute growth figures. These post-shock shifts reveal significant rebalancing, with patterns that persist for several days and frequently signal deeper adjustments rather than fleeting reactions.
Overall, these insights offer powerful predictive cues that can be integrated into risk management and trading strategies, enabling traders and institutions to anticipate market turns and navigate Bitcoin’s inherent unpredictability. The importance of early warning signals and persistent post-shock behavior cannot be overstated, as they provide a critical edge in decision-making. For more information and to explore these insights further, please get in contact with Amberdata.
Disclaimers
The information contained in this report is provided by Amberdata solely for educational and informational purposes. The contents of this report should not be construed as financial, investment, legal, tax, or any other form of professional advice. Amberdata does not provide personalized recommendations; any opinions or suggestions expressed in this report are for general informational purposes only.
Although Amberdata has made every effort to ensure the accuracy and completeness of the information provided, it cannot be held responsible for any errors, omissions, inaccuracies, or outdated information. Market conditions, regulations, and laws are subject to change, and readers should perform their own research and consult with a qualified professional before making any financial decisions or taking any actions based on the information provided in this report.
Past performance is not indicative of future results, and any investments discussed or mentioned in this report may not be suitable for all individuals or circumstances. Investing involves risks, and the value of investments can go up or down. Amberdata disclaims any liability for any loss or damage that may arise from the use of, or reliance on, the information contained in this report.
By accessing and using the information provided in this report, you agree to indemnify and hold harmless Amberdata, its affiliates, and their respective officers, directors, employees, and agents from and against any and all claims, losses, liabilities, damages, or expenses (including reasonable attorney’s fees) arising from your use of or reliance on the information contained herein.
Copyright © 2025 Amberdata. All rights reserved.
Michael Marshall
Mike Marshall is Head of Research at Amberdata. He leads pioneering research initiatives at the forefront of blockchain and cryptocurrency analytics. Mike is a seasoned quantitative analyst with a 15-year track record in developing AI-driven trading algorithms and pioneering proprietary cryptocurrency strategies. His...