DeFi Activity, Stablecoins, and the Impact on Price Volatility: Part 3
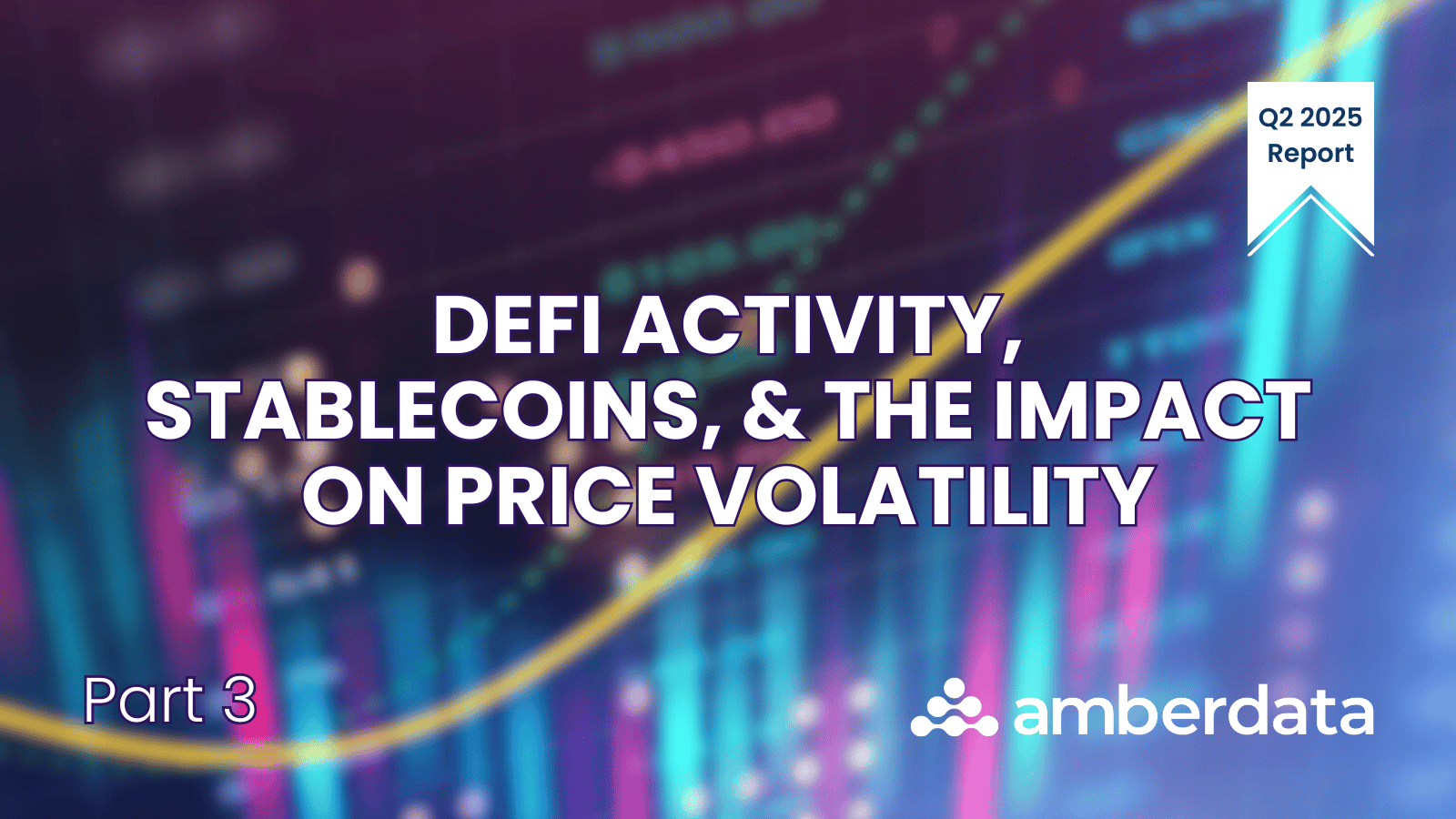
In our previous posts, we explored how stablecoins like USDC, USDT, and DAI are essential to the decentralized finance (DeFi) ecosystem. We showed how activities like lending and borrowing these stablecoins closely relate to Ethereum's (ETH) price movements. Part 1 focused on explaining how stablecoins work, including their collateral systems and liquidity trends. Part 2 went deeper, using advanced methods and real-time Amberdata data to study Ethereum volatility more closely.
Now, in this post, we take things a step further by looking at the timing of DeFi activities, such as loan repayments, withdrawals, and new borrowing. We're particularly interested in whether past activities in these protocols can predict future ETH volatility. Additionally, we examine special "shock days"—days when the number of repayments spikes significantly—to see how these extreme events impact the market.
Our findings provide practical insights for traders, investors, and those managing risk. For example, if there's a sudden increase in repayments (something Amberdata's data captures very well), it might be a signal that Ethereum’s volatility could soon rise. Recognizing these early signs helps traders adjust their strategies, manage risks better, and make more informed decisions. Additionally, understanding these dynamics can help DeFi protocols improve their systems, like adjusting fees or enhancing their risk models.
To uncover these relationships, we looked at how closely past DeFi activities relate to current ETH price volatility. We found clear connections, especially around repayment spikes. In simple terms, detailed DeFi data combined with careful analysis gives market participants powerful tools to predict volatility, stay ahead of market trends, and navigate DeFi with greater confidence.
For more detailed research and analysis, visit our research blog. For access to actionable market data and to gain deeper insights into the rapidly evolving DeFi landscape, get in touch with Amberdata today.
Lagged Variable Analysis
USDC
The analysis of lagged USDC variables demonstrates clear connections between recent lending activities and next-day Ethereum (ETH) volatility. Unlike DAI, which relies on decentralized crypto-collateralization, and USDT, which features less transparent reserve practices, USDC's institutional trust and fiat-backed transparency contribute uniquely to its observed liquidity dynamics. These differences help explain USDC's distinctive volatility signals.
- Yesterday's repayment count (numberOfRepays_lag1) correlates at 0.29 with today's volatility, indicating that rapid repayments reflect proactive institutional risk adjustments that precede volatility spikes.
- Withdrawal amounts from yesterday (withdrawnUSD_lag1) have a correlation of 0.25, suggesting large fund withdrawals signal potential liquidity stress or a shift towards safety, affecting market stability.
- Withdrawal frequency (numberOfWithdraws_lag1) correlates at 0.23, reinforcing that transaction size rather than mere frequency predominantly influences volatility.
Correlations weaken as the lag increases (repayments drop to 0.21 at two days and 0.17 at three days), highlighting that recent activities hold the strongest predictive power. High inter-correlations between consecutive days’ repayments (0.61) suggest persistent institutional behaviors, but also highlight potential multicollinearity issues in modeling. Overall, immediate recent repayments and withdrawals offer crucial early signals for anticipating ETH volatility, particularly valuable for institutional traders and risk managers actively monitoring USDC activities.
USDT
The analysis of lagged USDT variables highlights the significance of recent lending activities in forecasting next-day Ethereum (ETH) volatility. Compared to USDC, which benefits from institutional transparency, and DAI, characterized by decentralized collateralization, USDT occupies a distinct position due to its mixed institutional and retail user base and less transparent reserve management. These factors uniquely shape USDT’s volatility dynamics.
- Yesterday’s repayment count (numberOfRepays_lag1) exhibits a robust correlation of 0.31 with today's volatility, suggesting rapid repayments reflect significant shifts in risk perception or rebalancing activities.
- Total repayment amounts from yesterday (repaidUSD_lag1) correlate at 0.21, indicating that larger repayment values also provide meaningful volatility signals, though slightly less prominently than repayment counts.
- Withdrawal amounts from yesterday (withdrawnUSD_lag1) correlate at 0.18, reflecting that substantial withdrawals contribute notably to market instability.
These correlations diminish for activities occurring two or three days earlier (approximately 0.23), emphasizing recent activities' predictive strength. Notably high correlations between repayment counts and amounts (0.67) signal persistent behaviors within USDT lending markets, posing potential multicollinearity challenges for predictive modeling. Overall, immediate recent repayments and withdrawals within the USDT ecosystem serve as valuable early indicators of rising ETH volatility, critical for both institutional and retail market participants in effective risk management and strategic positioning.
DAI
The analysis of lagged DAI variables highlights how recent lending activities significantly influence next-day Ethereum (ETH) volatility. Unlike USDC, characterized by strong institutional transparency, and USDT, noted for its mixed institutional-retail dynamics, DAI uniquely stands out through its decentralized, crypto-collateralized model and cautious, incremental user behavior. This decentralized structure influences its volatility signals distinctively.
- Yesterday’s repayment count (numberOfRepays_lag1) strongly correlates at 0.32 with today's volatility, indicating rapid repayments reflect strategic risk adjustments driven by decentralized decision-making.
- Yesterday’s withdrawal count (numberOfWithdraws_lag1) correlates at 0.21, highlighting that increased withdrawal activity signals emerging market stress within the decentralized framework.
- Borrowing activity (numberOfBorrows_lag1) shows a weaker correlation of 0.14, emphasizing repayments and withdrawals as more direct indicators of market sentiment shifts.
Similar to USDC and USDT, correlations weaken over longer periods (repayment correlations decline to 0.24 and 0.23 at two- and three-day lags, respectively). Moderate inter-correlations (approximately 0.50 between consecutive days' repayments) indicate persistent behaviors typical of the DAI ecosystem, raising potential multicollinearity concerns for predictive models. Overall, immediate past repayments and withdrawals within the DAI protocol provide valuable early indicators of ETH volatility, particularly useful for risk managers navigating decentralized market dynamics.
Shock Days
This section explores "shock days," periods of exceptional stress marked by extreme repayment activity, where daily repayments exceed the 95th percentile historically. Identifying these shock days isolates significant market disturbances from regular activity, allowing us to analyze their distinct impact on Ethereum (ETH) volatility.
To pinpoint shock days, we calculate the 95th percentile threshold for repayments for each stablecoin. Days surpassing this threshold indicate notable market stress or liquidity shifts. Using an event-window analysis—examining ETH volatility three days before and after shock days—we capture systematic volatility patterns surrounding these extreme events.
This approach has two key benefits:
- Early Volatility Warnings: High repayments serve as early signals of imminent volatility spikes, alerting traders and risk managers to proactively adjust strategies.
- Enhanced Risk Management: Analyzing volatility dynamics before, during, and after shock days provides insights into stress duration and intensity, aiding in strategic decision-making.
Overall, examining shock days deepens our understanding of how extreme protocol activities influence ETH volatility, equipping market participants with valuable indicators to effectively manage periods of heightened market turbulence.
USDC
In our USDC dataset, we identified 55 shock days—days when repays exceeded the 95th percentile. Examining the event window for these shock days reveals a clear pattern in ETH volatility. Three days before a shock day, average volatility is relatively low at 0.03406, suggesting calm market conditions. Two days prior, volatility rises to 0.03942, and one day before it reaches 0.05059. On the shock day itself, volatility jumps to 0.06335, marking a significant market reaction as extreme USDC repays occur.
These findings reinforce our earlier observations from Parts 1 and 2, where USDC lending metrics—shaped by significant repays and large deposit flows—reflected shifts in institutional behavior and liquidity. The spike in volatility on the shock day implies that sudden, high levels of repays prompt rapid adjustments in market sentiment, which then translate into higher ETH price fluctuations.
Following the shock, the market appears to begin its recovery: volatility decreases to 0.05355 on day +1, drops to 0.04726 on day +2, and further settles at 0.04397 by day +3. This gradual normalization indicates that while extreme repays trigger a pronounced, albeit temporary, surge in volatility, the impact dissipates relatively quickly as market conditions stabilize.
This volatility pattern is evident when compared to regular market conditions. Volatility on USDC shock days (0.0634) is approximately 2.6 times higher than on typical trading days (0.0248), demonstrating that periods of elevated repayments in the USDC lending market closely correspond with increased Ethereum volatility. The quick normalization afterward suggests a relatively efficient market response within the USDC ecosystem.
USDT
In our USDT dataset, we identified 55 shock days—days when the number of repays exceeded the 95th percentile. Examining the event window for these shock days reveals a clear build-up and subsequent decline in ETH volatility. Three days before a shock day, average volatility is low at 0.03363, indicating relatively calm conditions. Two days prior, volatility rises to 0.04157, and one day before it reaches 0.04989. On the shock day itself, volatility jumps to 0.06417, demonstrating a marked market reaction when extreme repays occur.
These findings align with earlier USDT results, where we observed that repays are a key driver in influencing volatility. The surge in volatility on the shock day suggests that when USDT repays hit extraordinary levels, market participants may be rapidly adjusting their positions—whether by rebalancing or liquidating exposures—in response to perceived risks. Following the shock, volatility decreases to 0.05629 on day +1, drops further to 0.04805 on day +2, and settles at 0.04574 by day +3. This gradual normalization implies that the market absorbs the shock relatively quickly after the initial spike.
This volatility pattern becomes clearer when compared to typical market conditions. Volatility on USDT shock days (0.0642) is about 2.2 times higher than on regular days (0.0292), indicating that periods of elevated repayments in USDT lending coincide with increased Ethereum volatility. The relatively quick normalization following these shocks aligns with the mixed retail and institutional nature of the USDT market.
DAI
In our DAI dataset, we identified 54 shock days—days when the number of repays exceeds the 95th percentile. Examining the event window for these shock days reveals a clear build-up in volatility. Three days before a shock day, average volatility is 0.03611, rising to 0.0446 on day –2 and 0.0560 on day –1. On the shock day itself, volatility peaks at 0.0708, clearly marking the impact of extreme repay activity.
What is particularly noteworthy in the DAI system is the slower recovery. Unlike USDC or USDT, where volatility tends to normalize more quickly, the elevated volatility in DAI persists for a longer period. On day +1, volatility declines modestly to 0.0589, then to 0.0530 on day +2, and further to 0.0525 on day +3. Although there is a downward trend, the level at day +3 remains significantly higher than the pre-shock values.
This prolonged period of heightened volatility may reflect the unique characteristics of the DAI ecosystem, which is driven by a decentralized user base that tends to make cautious, incremental adjustments. The sustained impact suggests that extreme repays in DAI not only cause a sharp increase in volatility but also lead to a more gradual return to normal conditions, highlighting a distinctive dynamic in market behavior associated with DAI.
This volatility pattern is clearer when compared with typical market conditions. Volatility on DAI shock days (0.0708) is approximately 2.4 times higher than on regular trading days (0.0290), indicating that periods of elevated repayments in the DAI ecosystem correspond to notable increases and prolonged durations of Ethereum volatility. This finding highlights how decentralized user behaviors, characterized by gradual adjustments, can extend market instability following extreme events.
Conditional Probability Analysis
Conditional probability quantifies how the likelihood of volatility spikes increases during periods of extreme protocol activity. We define a volatility spike as days when ETH volatility exceeds its 95th percentile, typically occurring about 5% of the time. By comparing this baseline probability to volatility spikes on "shock days"—days with repayments above the 95th percentile—we clearly illustrate how extreme lending activity influences market volatility.
- USDC: The baseline probability of an ETH volatility spike is roughly 5.05%, but on shock days, this probability surges to approximately 41.82%, an eightfold increase. This significant rise indicates extreme USDC repayment activity is a strong predictor of increased volatility, reflecting institutional liquidity stress and rapid shifts in market sentiment.
- USDT: For USDT, the baseline spike probability is about 5.04%, rising to 34.55% on shock days. While slightly lower than USDC, this still substantial increase reflects the mixed institutional and retail nature of USDT activity, emphasizing the importance of repayment surges as early indicators of volatility.
- DAI: The baseline spike probability for DAI is approximately 5.04%, increasing significantly to 40.74% on shock days. This elevation aligns closely with USDC and highlights that extreme repayment activity in the decentralized DAI ecosystem strongly signals heightened market volatility, influenced by cautious, incremental adjustments typical of its user base.
Overall, extreme repayment activity markedly raises the likelihood of volatility spikes across all stablecoin protocols, providing traders and risk managers with critical early warnings to anticipate and manage market turbulence.
Conclusion
Our comprehensive analysis of DeFi lending dynamics underscores the critical relationship between stablecoin activities—particularly repayments—and Ethereum (ETH) volatility. Each stablecoin analyzed (USDC, USDT, and DAI) exhibits distinct characteristics influencing its role in volatility dynamics. USDC, known for its transparent fiat-backed reserves and institutional trust, predominantly serves institutional participants, clearly signaling shifts in liquidity and risk perception. USDT, widely adopted by both institutional and retail investors despite less transparency regarding its reserves, often reflects mixed market sentiment, resulting in varied responses during volatility events. DAI, distinguished by its decentralized crypto-collateralization and governance, is characterized by incremental, cautious adjustments driven by community behavior, leading to more gradual but significant market impacts.
Our conditional probability analysis demonstrates that extreme repayment events ("shock days") notably amplify volatility risk across all stablecoins, with spike probabilities surging approximately 2.2 to 2.6 times above typical trading conditions. The close alignment of these shock days with notable real-world events reinforces the systemic nature of such extreme repayment activities. For instance, the Celsius Network’s withdrawal freeze on June 13, 2022, triggered substantial repayments across all three stablecoins, reflecting widespread market panic and immediate risk-off behavior. Similarly, the March 2023 Silicon Valley Bank collapse coincided with significant repayment spikes, especially pronounced in USDC due to its direct exposure, temporarily causing it to lose its peg and amplifying market-wide volatility.
Other notable incidents—such as the onset of the Russia-Ukraine conflict in February 2022, the Terra-LUNA ecosystem collapse in May 2022, and FTX’s downfall in November 2022—also correspond closely with identified shock days. These correlations underscore how external crises consistently translate into heightened repayment activities, emphasizing their role as robust indicators of systemic stress rather than isolated protocol-specific reactions.
Moreover, our temporal analysis reveals a predictable volatility pattern surrounding shock events: a gradual increase in volatility leading up to the shock day, an acute volatility spike coinciding precisely with peak repayments, followed by gradual normalization over subsequent days. This recurring pattern provides market participants with actionable foresight, enabling proactive adjustments to trading and risk management strategies.
In summary, carefully monitoring stablecoin repayments emerges as essential for anticipating and managing ETH volatility. Understanding each stablecoin’s unique behaviors and their interactions with external market events enhances predictive capabilities, strengthens risk management frameworks, and positions stakeholders effectively to navigate periods of intense market turbulence.
Read more expert commentary and market research on our research blog. To leverage these insights and access actionable data for your trading and risk management strategies, connect with Amberdata today.
Disclaimers
The information contained in this report is provided by Amberdata solely for educational and informational purposes. The contents of this report should not be construed as financial, investment, legal, tax, or any other form of professional advice. Amberdata does not provide personalized recommendations; any opinions or suggestions expressed in this report are for general informational purposes only.
Although Amberdata has made every effort to ensure the accuracy and completeness of the information provided, it cannot be held responsible for any errors, omissions, inaccuracies, or outdated information. Market conditions, regulations, and laws are subject to change, and readers should perform their own research and consult with a qualified professional before making any financial decisions or taking any actions based on the information provided in this report.
Past performance is not indicative of future results, and any investments discussed or mentioned in this report may not be suitable for all individuals or circumstances. Investing involves risks, and the value of investments can go up or down. Amberdata disclaims any liability for any loss or damage that may arise from the use of, or reliance on, the information contained in this report.
By accessing and using the information provided in this report, you agree to indemnify and hold harmless Amberdata, its affiliates, and their respective officers, directors, employees, and agents from and against any and all claims, losses, liabilities, damages, or expenses (including reasonable attorney’s fees) arising from your use of or reliance on the information contained herein.
Copyright © 2025 Amberdata. All rights reserved.
Michael Marshall
Mike Marshall is Head of Research at Amberdata. He leads pioneering research initiatives at the forefront of blockchain and cryptocurrency analytics. Mike is a seasoned quantitative analyst with a 15-year track record in developing AI-driven trading algorithms and pioneering proprietary cryptocurrency strategies. His...